Principal Investigator
:
Prof. Dr hab. Rafał Weron
Wroclaw University of Science and Technology
Panel: HS4
Funding scheme
: MAESTRO 10
announced on
15 June 2018
Electricity price forecasting is a branch of forecasting at the intersection of economics/statistics, computer science and electrical engineering that focuses on predicting prices in wholesale electricity markets. Its origins date back to the early 1990s, when power sector deregulation led to the introduction of competitive markets in the UK and Scandinavia. The changes spread quickly, and today in many countries worldwide electricity is traded under market rules.
Prof. Rafał Weron, photo by Michał Łepecki
Over the last 25 years, a variety of methods and ideas have been tried for forecasting electricity prices. However, the unprecedented expansion of renewable generation and active demand side management on one hand, and machine learning advances as well as increases in computing power on the other, have provided the impetus and - much-needed - technical opportunities to cross the frontiers of today's electricity price forecasting.
It is the aim of the CrossFIT project to go beyond the state-of-the-art and bring electricity price forecasting to a new level. The research is being carried out in international collaboration (Germany, the Netherlands, USA) along four directions.
The first is based on recent advances in deep learning (i.e., the use of multilayer neural nets) and statistical learning (e.g., regularization algorithms that penalize overfitting and favor parsimonious models) to provide better performing models, able to extract useful information in the era of Big Data (i.e. big and complex data sets). In the project, we have already developed the LQRA model, which uses LASSO method to automatically select variables in quantile regression, thus solving the problem of the method's vulnerability to low quality predictors. In collaboration with researchers from Carnegie Mellon, we have also developed the NBEATSx deep neural network architecture, which allows for the interpretability of its components. The latter is of great practical importance and in line with the "eXplainable AI" (XAI) trend.
Prof. Rafał Weron, photo by Michał Łepecki
The second direction uses the latest concepts of averaging forecasts of the same or different models across a set of calibration windows. In collaboration with researchers from the Delft University of Technology, we have developed a set of best practices and provided open-access Python (one of the most popular programming languages) codes for two competitive benchmarks that use averaging, so that new algorithms can be rigorously evaluated in future studies. In addition, in collaboration with researchers from the University of Duisburg-Essen, we have tested new approaches to averaging probabilistic forecasts, i.e., forecasts of entire distributions.
The third direction concerns trajectory (or ensemble) forecasts, where the difficulty lies mainly in the complexity of the econometric apparatus needed to develop forecasting models and tools for assessing these de facto multivariate predictions. In the project, we have proposed methods that make use of copulas (high-dimensional distribution function used to describe the dependance between random variables) to transform probabilistic into trajectory forecasts.
Finally, the fourth direction concerns looking at prediction errors from a completely different perspective. The focus is not on the errors themselves, but on the financial impact of these errors. The universal trading strategies developed in the project make it possible to assess what is really important for companies. In particular, we were able to show that strategies based on probabilistic forecasts lead to much higher profits than those based on point forecasts and, interestingly, can achieve profits that are very close to the theoretical maximum — the "oracle" strategy, according to which everything is always bought at the lowest price of the day and sold at the highest.
For more information on the project, visit the website of the Department of Operations Research and Business Intelligence.
Project title: Crossing Frontiers in electricity prIce forecasTing (CrossFIT)
Prof. Dr hab. Rafał Weron
Professor of Management Science, member of the Academia Europaea, and Head of the Department of Operations Research and Business Intelligence at the Wrocław University of Science and Technology. He is one of the leading world experts in energy forecasting.
He introduced Quantile Regression Averaging (QRA) into the literature and business practice, which was later used by two winning teams in the Global Energy Forecasting Competition 2014, and the interpretable NBEATSx deep neural net architecture for forecasting electricity prices, which was named one of Deep Tech's top five development directions by Forbes magazine in 2023.
But perhaps his most important achievement is a "series" of three review articles on electricity price forecasting that promote rigorous use of methods, error metrics, and statistical tests. The 2014 article received the Emerald Citation of Excellence Award and the International Institute of Forecasters Award, and all three are listed as Highly Cited Papers in the Web of Science.
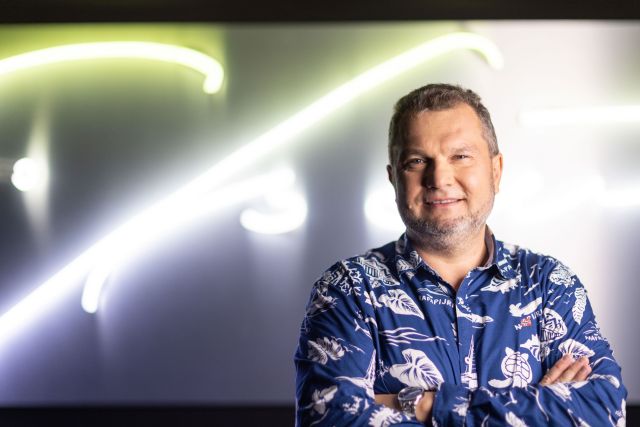